Recently, the center published an article "Strategies for training optical neural networks" in National Science Open. The article systematically summarizes the current mainstream optical neural network training strategies for the first time, analyzes the advantages and disadvantages of different strategies, and finally summarizes and looks forward to the bottlenecks currently encountered in the training of optical neural networks as well as the future development direction.
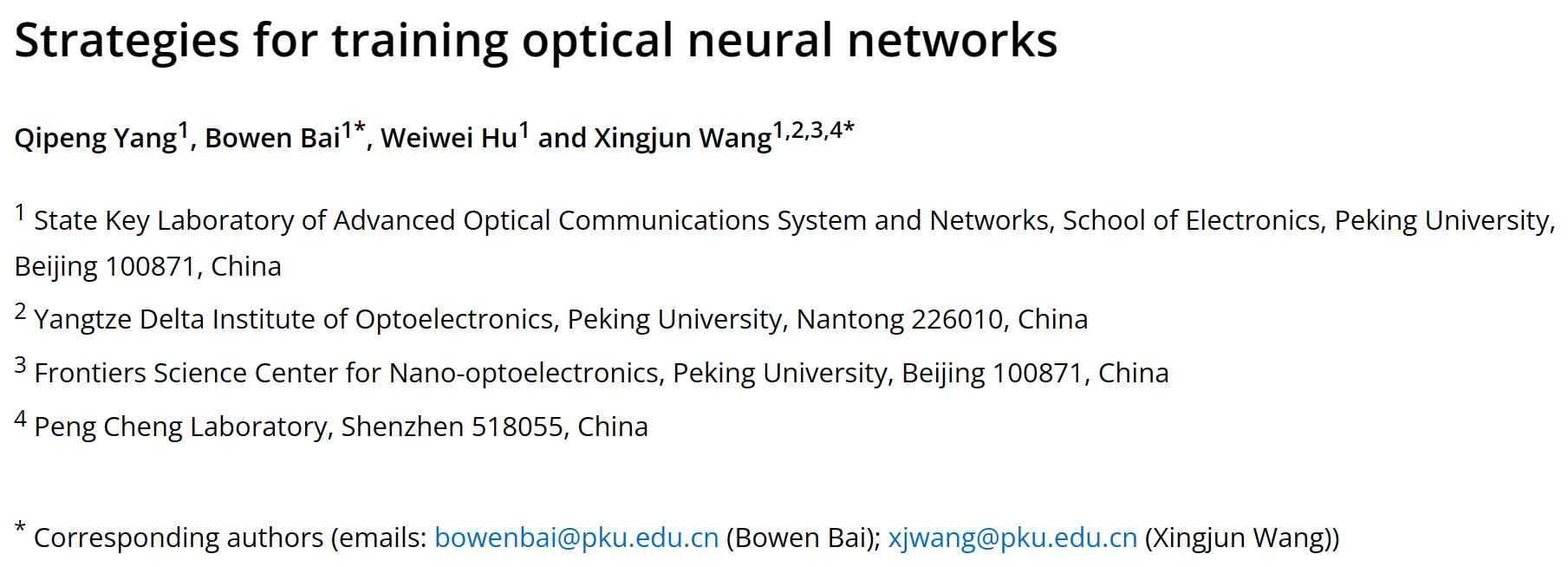
Fig. 1 Screenshot of the paper
Deep learning techniques have produced important roles in computer vision, natural language processing and other fields in recent years. With the advent of the post-Moore era, traditional microelectronic processors have struggled to meet the huge demand for high computing power and low power consumption for deep learning tasks. Benefiting from photon's large bandwidth and ultra-high speed, using it to implement deep learning tasks can achieve a huge increase in computational energy efficiency and speed. As a result, neural network accelerators implemented based on specific optical structures are widely regarded as the next generation of dedicated computing hardware for deep learning.
Currently, optical neural networks have been implemented for tasks such as vowel recognition and handwritten digit recognition. However, when faced with more complex computational tasks, such as end-to-end target detection, photonic neural networks struggle. One reason is that current mainstream optical architectures have difficulty in realizing ultra-large-scale neuron deployment, limiting the expressive power of neural networks. This problem can be solved by future novel architecture design and optical neuron multiplexing. And another more dominant reason is the lack of large-scale and efficient and fast training strategies for optical neural networks. Unlike the universality of backpropagation in traditional training strategies, optical neural networks differ in their training strategies depending on the structure of the optical realization. There are three mainstream training strategies. The first in-silico strategy relies on mapping pre-trained weight data to optical neural networks by means of a look-up table, but this approach is not robust and is prone to failure due to environmental changes. In order to improve the robustness of the training, the second type of strategy adopts in-situ training. By using gradient-based and gradient-free algorithms, the optical neural network is better at resisting the adverse effects caused by changes in the external environment as well as process errors. However, this type of training strategy lacks a mechanism for obtaining internal information and faces certain difficulties in terms of algorithm convergence speed. The third category combines in-silico and in-situ training strategies to solve the problem of difficult access to internal gradient information. However, because it uses a neural network model in the electrical domain, this training strategy can only work on the inference side of the optical neural network. In order to solve the current problem that training strategies cannot be deployed on a large scale and are not efficient, the mechanism of rapid access to information within the system and the architectural design of hardware and software collaboration need to be emphasized in the future.
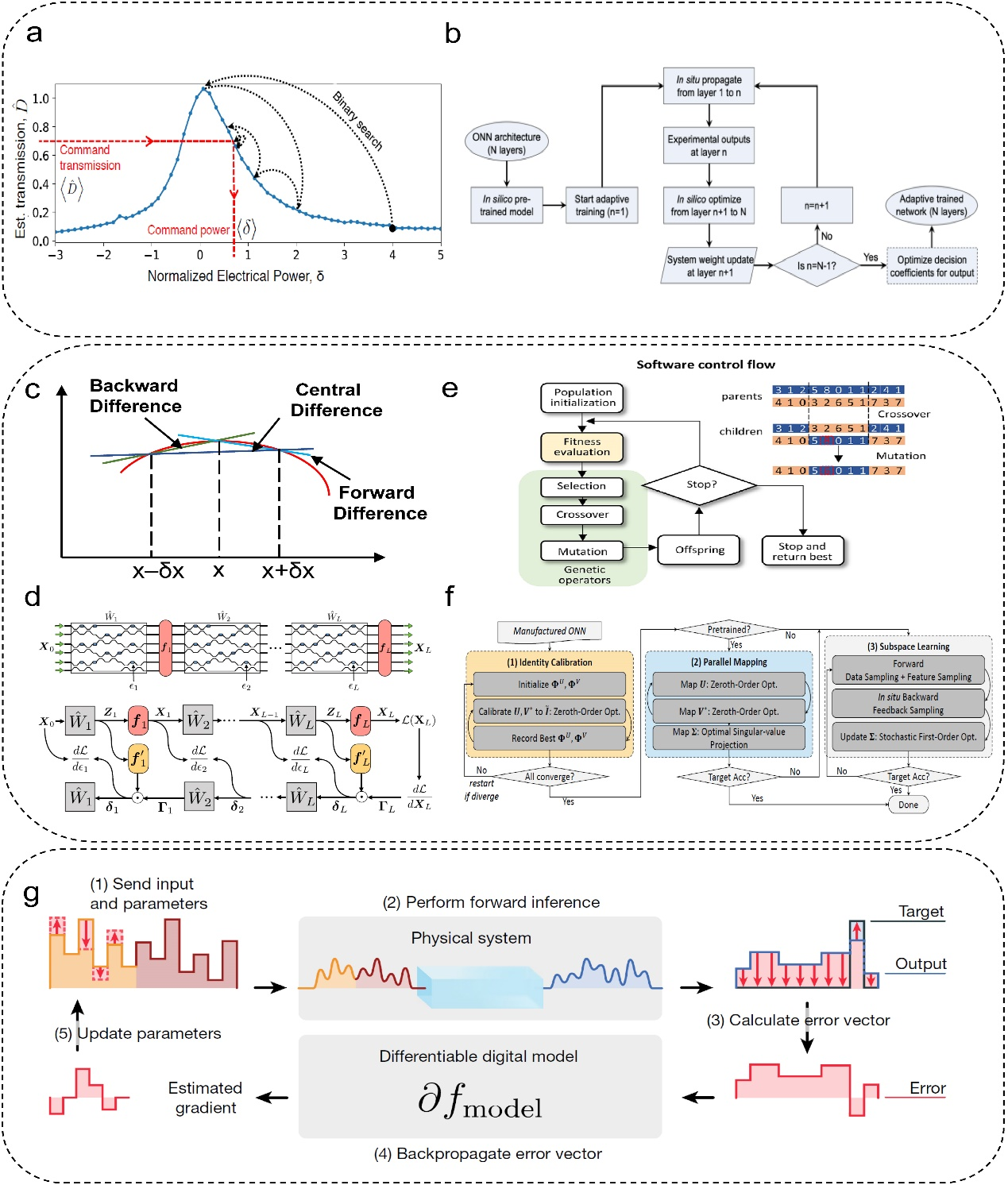
Fig. 2 Flowchart of different optical neural network training strategies
The first author of the paper is Qipeng Yang, a PhD student in the class of 2020. Corresponding authors are Prof. Xingjun Wang of the School of Electronics, and Bowen Bai, a postdoctoral fellow at the Center for Boya. The main co-authors also include Prof. Vivian Hu of the School of Electronics. This work was done by the State Key Laboratory of Regional Optical Fiber Communication Networks and Novel Optical Communication Systems, School of Electronics, Peking University as the first unit
Link to the original paper:
https://www.nso-journal.org/articles/nso/full_html/2022/03/NSO-2022-0042/NSO-2022-0042.html