On January 5, 2023, the Center published the article "Microcomb-based integrated photonic processing unit" online in Nature Communications. The article reports an ultra-high arithmetic density silicon-based integrated photonic processor based on photonic neural networks for the first time. The research team spent three years to overcome two worldwide technical challenges, namely, the calibration of multiwavelength parallel optical computing systems and ultra-high-precision weight loading.
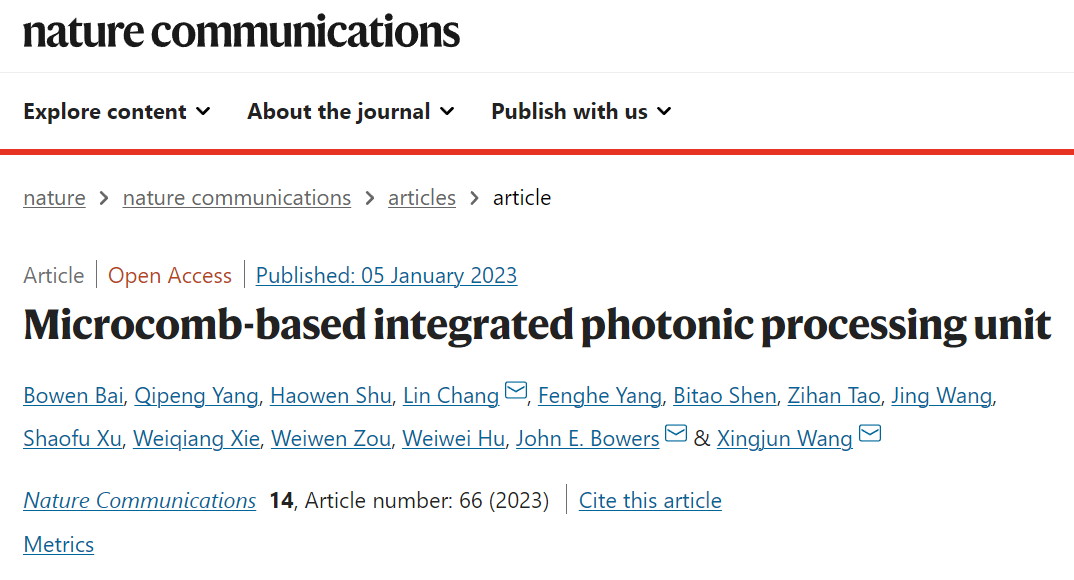
Fig. 1 Screenshot of the paper
Artificial Intelligence (AI) techniques have previously been widely used in data-intensive computing tasks. In the post-Moore era, photonic neural networks have emerged to meet the huge demand for large computing power and low energy consumption in AI. Basic mathematical operations such as matrix multiplication and convolution in neural networks can be realized using photons in specific optical structures. Thanks to the natural advantages of photonic ultra-high speeds, large bandwidths and low energy consumption, computing speeds and energy efficiency are dramatically increased. In recent years, photonic neural networks have become realizable through mature optoelectronic integration platforms. Due to its tremendous advantages of small size, scalability, and low power consumption, this new type of photonic processing unit is expected to break through the arithmetic and energy-efficiency bottlenecks of traditional microelectronic processors, while revolutionizing the deployment of hardware for artificial intelligence.
In order to further exploit the optical computing potential, photonic neural networks need to boost the computational throughput through parallelism. The introduction of WDM in photonic neural networks is an effective tool. However this advanced computational architecture faces great challenges in terms of integration. In addition to the difficulty of realizing an integrated multi-wavelength light source, another important difficulty is that multi-wavelength photonic neural networks are more complex compared to single-wavelength scheme systems, requiring finer calibration methods and more effective control. Therefore, for a long time, siliconization has been a bottleneck restricting the development of parallel photonic neural networks, hindering the promotion and popularization of related technologies.
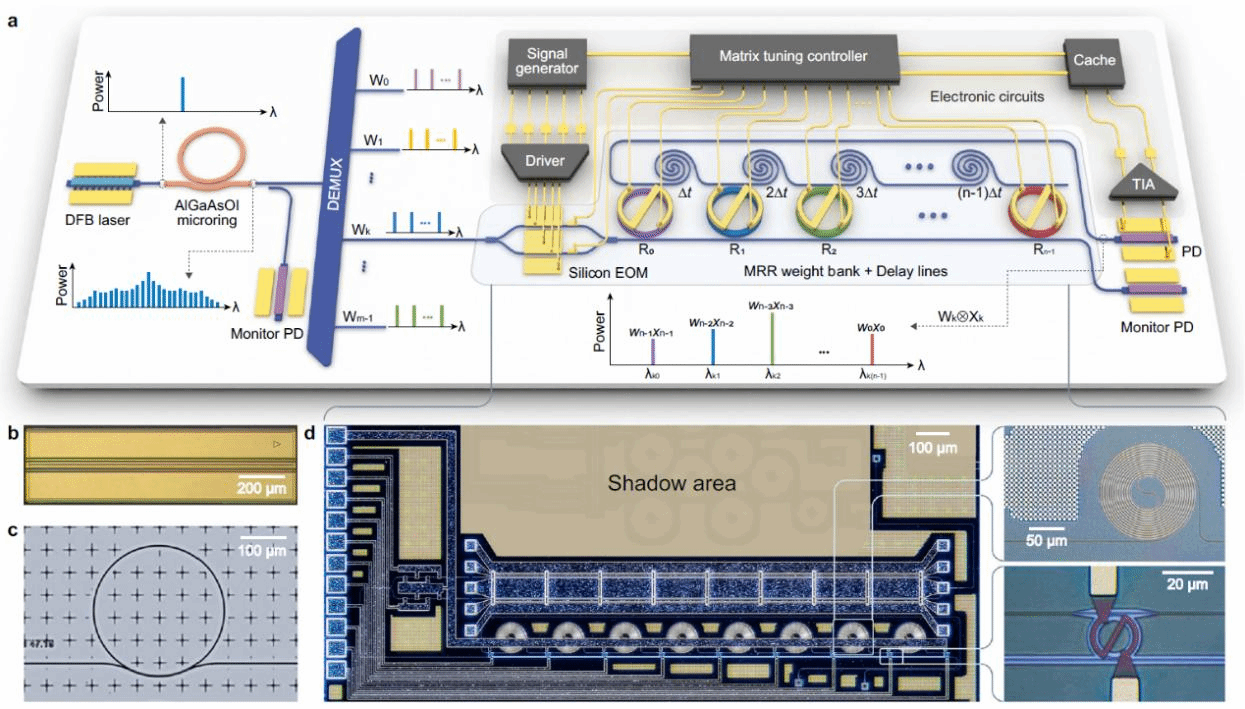
Fig. 2 High-computer-density integrated photonic processor
In this work, the research team realized a silicon-based integrated photonic processor consisting of a multi-wavelength light source, a high-speed data loading/receiving unit and a computational core. The calibration method developed for the multi-wavelength computing system enables accurate tuning of each part of the optoelectronic device and lays the foundation for the realization of the highest weighting control accuracy (9 bits) available. The optoelectronic processor has an arithmetic density of up to 1.04 TOPS/mm2, thanks to extremely high system integration and ultra-high-speed information loading/receiving rates. In the image edge detection and handwritten digit recognition test tasks, the quality of edge detection and the accuracy of recognition (96.6%) were comparable to electronic computers (97.0%). This work points the way to the realization of fully integrated multi-wavelength optical computing systems and is expected to provide new solutions for arithmetic breakthroughs in the entire field of artificial intelligence in the future.
The paper's co-first authors are Bowen Bai, a Boya postdoctoral fellow at the Center; Qipeng Yang, a Ph.D. student in the class of 2020; Haowen Shu, a Boya postdoctoral fellow; and Assistant Professor Chang Lin of the College of Electronics. Prof. Xingjun Wang, Prof. John E. Bowers of the University of California, Santa Barbara, and Assistant Prof. Chang Lin of the School of Electronics are the corresponding authors of the paper. Key collaborators also include Prof. Wei-Wen Zou of Shanghai Jiao Tong University, Associate Researcher Feng-He Yang of Zhangjiang Laboratory, Dr. Wei-Qiang Xie of the University of California, Santa Barbara (now an Associate Professor at Shanghai Jiao Tong University), Prof. Wei-Wei Hu of the School of Electronics, and the center's Ph.D. students in the Class of 2019, Bitao Shen, and in the Class of 2020, Zihan Tao. This work was completed by the State Key Laboratory of Regional Optical Fiber Communication Networks and Novel Optical Communication Systems, School of Electronics, Peking University, as the first unit, and is also an important achievement of the cooperation between the Beijing and Shanghai branch campuses of the State Key Laboratory.
Link to the original paper:
https://www.nature.com/articles/s41467-022-35506-9